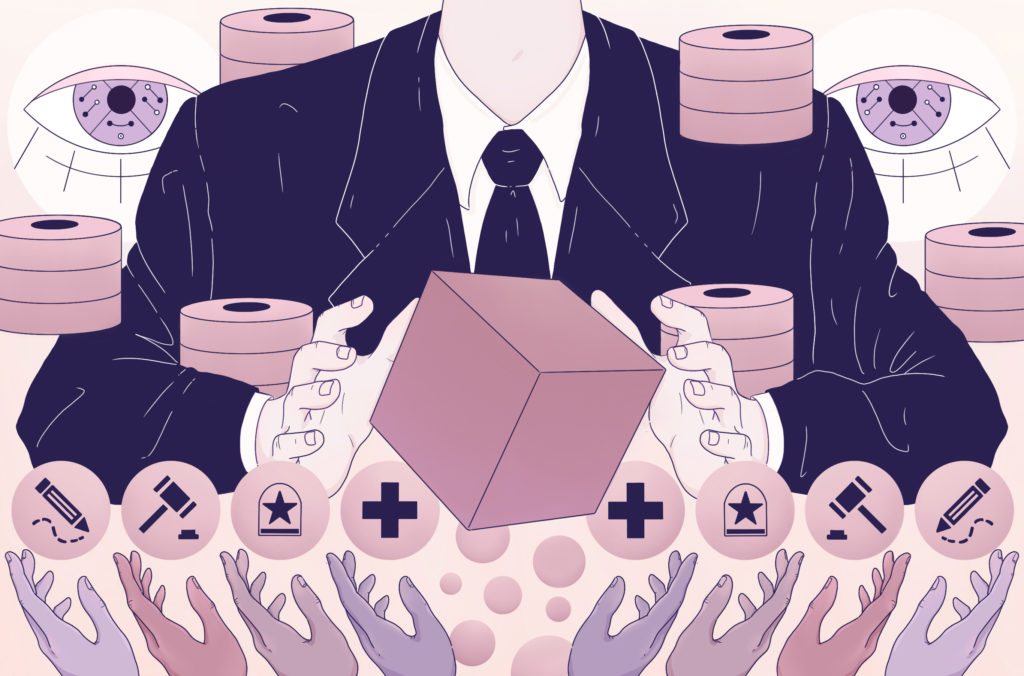
By Paz Pena and Joana Varon.
Artificial Intelligence (A.I.) is a discipline that aims to create machines that simulate cognitive functions, such as learning or problem-solving. It is called artificial because, unlike natural intelligence, common to humans or other living beings, which involves consciousness and emotions, it is displayed by machines through computational processing. Its definition can include a wide variety of methods and tools, such as machine learning (ML), facial recognition, speech recognition, etc. Machine Learning is the field most commonly associated with A.I. and refers to a method of data analysis that automates analytical models by identifying patterns that give machines the ability to “learn” from data without being explicitly given instructions on how to do so.
According to the “Algorithmic Accountability Policy Toolkit”, released by AINOW, A.I. should be understood as a development from the dominant social practices of the engineers and computer scientists who design the systems, the industrial infrastructure, and the companies that run these systems. Therefore, “a more complete definition of A.I. includes technical approaches, social practices, and industrial power.” (AINOW, 2018).
A.I. in the public sector
AI systems are based on models that are abstract representations, universalizations, and simplifications of complex realities where much information is being left out according to the judgment of their creators. As Cathy O’Neil observes in her book “Weapons of Math Destruction”: “[M]odels, despite their reputation for impartiality, reflect goals and ideology. […] Our own values and desires influence our choices, from the data we choose to collect to the questions we ask. Models are opinions embedded in mathematics.” (O’Neil, 2016).
Hence, algorithms are fallible human creations. Humans are always present in the construction of automated decision systems: they determine the objectives and uses of the systems, they are the ones who determine what data should be collected for those objectives and uses, they collect that data, they decide how to train the people who use those systems, evaluate software performance, and ultimately act on decisions and evaluations made by systems.
More specifically, as Tendayi Achiume, Special Rapporteur on contemporary forms of racism, racial discrimination, xenophobia and related intolerance, poses in the report “Racial discrimination and emerging digital technologies”, databases used in these systems are the product of human design and can be biased in various ways, potentially leading to – intentional or unintentional – discrimination or exclusion of certain populations, in particular, minorities as based on racial, ethnic, religious and gender identity. (Tendayi, 2020).
Given these problems, it should be recognized that part of the technical community has made various attempts to mathematically define “fairness”, and thus meet a demonstrable standard on the matter. Likewise, several organizations, both private and public, have undertaken efforts to define ethical standards for AI. The data visualization “Principled Artificial Intelligence” (Berkman Klein, 2020) shows the diversity of ethical and human rights-based frameworks that emerged from different sectors from 2016 onwards with the goal to guide the development and use of AI systems. The study shows “a growing consensus around eight key thematic trends: privacy, accountability, safety and security, transparency and explainability, fairness and non-discrimination, human control of technology, professional responsibility and promotion of human values.” Nevertheless, as we can see from that list, none of this consensus is driven by social justice principles. Instead of asking how to develop and deploy an A.I. system, shouldn’t we be asking first “why to build it?”, “is it really needed?”, “on whose request?”, “who profits?”, “who loses?” from the deployment of a particular A.I. system? Should it even be developed and deployed?
Despite all these questions, many States around the world are increasingly using algorithmic decision-making tools to determine the distribution of goods and services, including education, public health services, policing, and housing, among others. Beyond principles, more empirically, particularly within the US, where some of these projects have been developed further than pilot phases, confronted with the evidence on bias and harm caused by automated decisions, Artificial Intelligence programs have faced criticism on several fronts. But more recently, governments in Latin America are also following the hype to deploy A.I. systems in public services, sometimes with the support of US companies that are using the region as a laboratory of ideas which, perhaps fearing criticism in their home countries, are not even tested in the US first.
With the goal to build a case-based anti-colonial feminist toolkit to question these systems from perspectives that go beyond criticism from the Global North, through desk research and a questionnaire distributed across digital rights networks in the region, we have mapped projects where algorithmic decisions making systems are being deployed by governments with likely harmful implications on gender equality and all its intersectionalities. Unlike many A.I. projects and policies that tend to depart from the start-up motto “move fast and break things”, our recollection of cases depart from the assumption: unless you don’t prove you are not causing harm, if your targets are marginalized communities, you are very likely to be.
As a result, until April 2021, we have mapped 24 cases in Chile, Brazil, Argentina, Colombia, and Uruguay, which we were able to classify into 5 categories: judicial system, education, policing, social benefits, and public health. Several of them are in an early stage of deployment or developed as pilots.
So far, we have endured a task to analyze possible harms by A.I. programs deployed in the areas of education and social benefits, in Chile, Argentina, and Brazil. As a result, based on both our bibliographic review and our case-based analysis, we are gradually expanding an empirically tested case-based framework to serve as one of the instruments for our feminist anti-colonial toolkit. Hopefully, it will be a scheme that can help us to pose structural questions about whether a given governmental A.I. system may incur possible harm to several feminist agendas.
Possible harms by algorithmic decision-making deployed in public policies
Next, we present a summary of criticisms that are being posed to A.I. systems deployed by the public sector, which intersect and feed on each other. Based on an overall bibliographical review and also finding from the case-based analysis, this is an attempt to create a work-in-progress framework of analysis that goes beyond the discourses of fairness, ethical or human-centric A.I. and seeks a holistic structure that considers power relations to question the idea of deploying A.I. systems in several helms of the public sector:

Oppressive A.I. Framework by Joana Varon and Paz Peña. Design by Clarote for notmy.ai
A. Surveillance of the poor: turning poverty and vulnerability into machine-readable data
The former United Nations Rapporteur on Extreme Poverty and Human Rights, Philip Alston, has criticized the phenomenon in which “systems of social protection and assistance are increasingly driven by digital data and technologies that are used to automate, predict, identify, surveil, detect, target and punish.” (A/74/48037 2019). “These granular data sources enable authorities to infer people’s movements, activities, and behavior, not without having ethical, political, and practical implications of how the public and private sector view and treat people”, according to Linnet Tylor, in her article “What is data justice?” (TYLOR, 2017), this is even more challenging in cases of low-income portions of the population, since the ability of authorities to collect accurate statistical data about them has been previously limited, but now is targeted by regressive classifications systems that profile, judge, punish and surveil.
Most of these programs take advantage of the tradition of State surveillance on vulnerable populations (Eubanks, 2018), turn their existence into data, and now use algorithms to determine the provision of social benefits by the States. Analyzing the case of the USA, Eubanks shows how the usage of A.I. system is subjected to a long tradition of institutions that manage poverty and that seek through these innovations to adapt and continue their urge to contain, monitor, and punish the poor. Therefore, turning poverty and vulnerability into machine-readable data, with real consequences on the lives and livelihoods of the citizens involved. (Masiero, & Das, 2019). Likewise, Cathy O’Neil (2016), analyzing the usages of AI in the United States (US), asserts that many A.I. systems “tend to punish the poor”, meaning it is increasingly common for wealthy people to benefit from personal interactions, while data from the poor are processed by machines making decisions about their rights.
This becomes even more relevant when we consider that social class has a powerful gender component. It is common for public policies to speak of the “feminization of poverty.” In fact, at the IV United Nations Conference on Women, held in Beijing in 1995, as a conclusion, it was stated that 70% of poor people in the world were women. The reasons why poverty affects women have to do not with biological reasons but with structures of social inequality that make it more difficult for women to overcome poverty, such as access to education and employment (Aguilar, 2011).
B. Embedded racism
For the UN Special Rapporteur, E. Tandayi (2020), emerging digital technologies should also be understood as capable of creating and maintaining racial and ethnic exclusion in systemic or structural terms. This is also what tech researchers on race and AI in the US, such as Ruha Benjamin, Joy Buolamwini, Timnit Gebru and Safiya Noble highlight in their case studies, but also in Latin America, Nina da Hora, Tarcisio Silva and Pablo Nunes, all from Brazil, have pointed out while investigating facial recognition technologies, particularly for policing. Ruha Benjamin (2019) discusses how the use of new technologies reflects and reproduces the existing racial injustices in US society, even though they are promoted and perceived as more objective or progressive than the discriminatory systems of an earlier era. In this sense, for this author, when AI seeks to determine how much people of all classes deserve opportunities, the designers of these technologies build a digital caste system structured on existing racial discrimination.
From technology development itself, in her research, Noble (2018) demonstrates how commercial search engines such as Google not only mediate but are mediated by a series of commercial imperatives that, in turn, are supported by both economic and information policies that end up endorsing the commodification of women’s identities. In this case, she exposes this by analyzing a series of Google searches where black women end up being sexualized by the contextual information the search engine throws up (e.g., linking them to wild and sexual women).
Another notable study is by Buolamwini & Gebru (2018), who analyzed three commercial facial recognition systems that include the ability to classify faces by gender. They found that the systems exhibit higher error rates for darker-skinned women than for any other group, with the lowest error rates for light-skinned men. The authors attribute these race and gender biases to the composition of the data sets used to train these systems, which were overwhelmingly composed of lighter-skinned male-appearing subjects.
C. Patriarchal by Design: sexism, compulsory heteronormativity, and gender binarism
Many AI systems work by sorting people into a binary view of gender, as well as by reinforcing outdated stereotypes of gender and sexual orientation. A recent study co-authored by DeepMind senior staff scientist Shakir Mohamed exposes how the discussion about algorithmic fairness has omitted sexual orientation and gender identity, with concrete impacts on “censorship, language, online safety, health, and employment” leading to discrimination and exclusion of LGBT+ people.
Gender has been analyzed in a variety of ways in Artificial Intelligence. West, Whittaker & Crawford (2019) argue that the diversity crisis in industry and bias issues in AI systems (particularly race and gender) are interrelated aspects of the same problem. Researchers commonly examined these issues in isolation in the past, but mounting evidence shows that they are closely intertwined. However, they caution that, despite all the evidence on the need for diversity in technology fields, both in academia and industry, these indicators have stagnated.
Inspired by Buolamwini & Gebru (2018), Silva & Varon (2021) researched how facial recognition technologies affect transgender people and concluded that, although the main public agencies in Brazil already use these types of technology to verify identities for accessing public services, there is little transparency on the accuracy of them (tracking false positives or false negatives), as well as on privacy and data protection in the face of data sharing practices between public administration agencies and even between private entities.
In the case of Venezuela, amid a sustained humanitarian crisis, the State has implemented biometric systems to control the acquisition of basic necessities, resulting in several complaints of discrimination against foreigners and transgender people. According to Díaz Hernández (2021), legislation to protect transgender people is practically nonexistent. They are not allowed recognition of their identity, which makes this technology resignify the value of their bodies “and turns them into invalid bodies, which therefore remain on the margins of the system and the margins of society” (p.12).
In the case of poverty management programs through big data and Artificial Intelligence systems, it is crucial to look at how poor women are particularly subject to surveillance by the States and how this leads to the reproduction of economic and gender inequalities (Castro & López, 2021).
D. Colonial extractivism of data bodies and territories
Authors like Couldry and Mejias (2018) y Shoshana Zuboff (2019) review this current state of capitalism where the production and extraction of personal data naturalize the colonial appropriation of life in general. To achieve this, a series of ideological processes operate where, on the one hand, personal data is treated as raw material, naturally disposable for the expropriation of capital and, on the other, where corporations are considered the only ones capable of processing and, therefore, appropriate the data.
Regarding colonialism and Artificial Intelligence, Mohamed et al. (2020) examine how coloniality presents itself in algorithmic systems through institutionalized algorithmic oppression (the unjust subordination of one social group at the expense of the privilege of another), algorithmic exploitation (ways in which institutional actors and corporations take advantage of often already marginalized people for the asymmetric benefit of these industries) and algorithmic dispossession (centralization of power in the few and the dispossession of many), in an analysis that seeks to highlight the historical continuities of power relations.
Crawford (2021) calls for a more comprehensive view of Artificial Intelligence as a critical way to understand that these systems depend on exploitation: on the one hand, of energy and mineral resources, of the cheap labor, and, in addition, of our data at scale. In other words, AI is an extractive industry.
All these systems are energy-intensive and heavily dependent on minerals, sometimes, extracted from areas where there is. In Latin America alone, we have the lithium triangle within Argentina, Bolivia and Chile, as well as several deposits of 3TG minerals (tin, tungsten, tantalum and gold) in the Amazon region, all minerals used in cutting edge electronic devices. As Danae Tapia and Paz Pena poses, digital communications are built upon exploitation, even though, “sociotechnical analyses of the ecological impact of digital technologies are almost non-existent in the hegemonic human rights community working in the digital context.” (Tapia & Pena, 2021) And, even beyond ecological impact, Camila Nobrega and Joana Varon also expose, the green economy narratives altogether with technosolutionisms are “threatening multiple forms of existence, of historical uses and collective management of territories”, not by chance the authors found out that Alphabet Inc., Google parent company is exploiting 3TG minerals in regions of the Amazon where there is a land conflict with indigenous people (Nobrega & Varon, 2021).
E. Automation of neoliberal policies
As Payal Arora (2016) frames it, discourses around big data have an overwhelmingly positive connotation thanks to the neoliberal idea that the exploitation for profit of the poor’s data by private companies will only benefit the population. From an economic point of view, digital welfare states are deeply intertwined with capitalist market logic and, particularly, with neoliberal doctrines that seek deep reductions in the general welfare budget, including the number of beneficiaries, the elimination of some services, the introduction of demanding and intrusive forms of conditionality of benefits, to the point that -as Alston has estated (2019)- individuals do not see themselves as subjects of rights but as service applicants (Alston, 2019, Masiero and Das, 2019). In this sense, it is interesting to see that IA systems, in their neoliberal efforts to target public resources, also classify who the poor subject is through automated mechanisms of exclusion and inclusion (López, 2020).
F. Precarious Labour
Particularly focused on artificial intelligence and the algorithms of Big Tech companies, anthropologist Mary Gray and computer scientist Siddharth Suri point out the “ghost work” or invisible labor that powers digital technologies. Labeling images, cleaning databases are all manual work very often performed in unsavory working conditions “to make the internet seem smart”. Communalities of these jobs are very precarious working conditions, normally marked by overwork, underpaid, with no social benefits or stability, very different from the work conditions of the creators of such systems (Crawford, 2021). Who takes care of your database? As always, care work is not recognized as valuable work.
G. Lack of Transparency
According to AINOW (2018), when government agencies adopt algorithmic tools without adequate transparency, accountability, and external oversight, their use can threaten civil liberties and exacerbate existing problems within government agencies. Along the same lines, OECD (Berryhill et al., 2019) postulates that transparency [on the part of] is strategic to foster public trust in the tool.
More critical views comment on the neoliberal approach when transparency depends on the responsibility of individuals, as they do not have the time or the desire to commit to more significant forms of transparency and consent online (Annany & Crawford, 2018). Thus, government intermediaries with special understanding and independence should play a role here (Brevini & Pasquale, 2020). Furthermore, Annany & Crawford (2018) suggest that what the current vision of transparency in AI does is fetishize the object of technology, without understanding that technology is an assembly of human and non-human actors, therefore, to understand the operation of AI it is necessary to go beyond looking at the mere object.
Case-based analysis:
Beyond prominent antiracist and feminist fellow scholars that are focused on the deployment of AI systems in the US, we want to contribute building feminists lenses to question AI system based in our Latin American experiences and inspired by decolonial feminist theories, hence, in the next post we will focus on analyzing cases from Latin America with these lenses:
a) Sistema Alerta Niñez – SAN, Chile
b) Plataforma Tecnológica de Intervención Social / Projeto Horus – Argentina and Brazil
Bibliography
Aguilar, P. L. (2011). La feminización de la pobreza: conceptualizaciones actuales y potencialidades analíticas. Revista Katálysis, 14(1),126-133
AINOW. 2018. Algorithmic Accountability Policy Toolkit. https://ainowinstitute.org/aap-toolkit.pdf
Alston, Philip. 2019. Report of the Special rapporteur on extreme poverty and human rights. Promotion and protection of human rights: Human rights questions, including alternative approaches for improving the effective enjoyment of human rights and fundamental freedoms. A/74/48037. Seventy-fourth session. Item 72(b) of the provisional agenda.
Ananny, M., & Crawford, K. (2018). Seeing without knowing: Limitations of the transparency ideal and its application to algorithmic accountability. New Media & Society, 20(3), 973–989. https://doi.org/10.1177/1461444816676645
Arora, P. (2016). The Bottom of the Data Pyramid: Big Data and the Global South. International Journal of Communication, 10, 1681-1699.
Benjamin, R. (2019). Race after technology: Abolitionist tools for the new Jim code. Polity.
Berryhill, J., Kok Heang, K., Clogher, R. & McBride, K. (2019). Hello, World: Artificial Intelligence and its Use in the Public Sector. OECD.
Brevini, B., & Pasquale, F. (2020). Revisiting the Black Box Society by rethinking the political economy of big data. Big Data & Society. https://doi.org/10.1177/2053951720935146
Bridle, J. (2018). New Dark Age: Technology, Knowledge and the End of the Future. Verso Books.
Bright, J., Bharath, G., Seidelin, C., & Vogl, T. M. (2019). Data Science for Local Government (April 11, 2019). Available at SSRN: https://ssrn.com/abstract=3370217 or http://dx.doi.org/10.2139/ssrn.3370217
Buolamwini, J. & Gebru, T. (2018). Gender Shades: Intersectional Accuracy Disparities in Commercial Gender Classification. Proceedings of the 1st Conference on Fairness, Accountability and Transparency, in PMLR 81:77-91Castro, L. & López, J. (2021). Vigilanco a las “buenas madres”. Aportes desde una perspectiva feminista para la investigación sobre la datificación y la vigilancia en la política social desde Familias En Acción. Fundación Karisma. Colombia.
Couldry, N., & Mejias, U. (2019). Data colonialism: rethinking big data’s relation to the contemporary subject. Television and New Media, 20(4), 336-349.
Crawford, K. (2021). Atlas of AI. Power, Politics, and the Planetary Costs of Artificial Intelligence. Yale University Press.
Díaz Hernández, M. (2020) Sistemas de protección social en Venezuela: vigilancia, género y derechos humanos. In Sistemas de identificación y protección social en Venezuela y Bolivia. Impactos de género y otros tipos de discriminación. Derechos Digitales.
Eubanks, V. (2018). Automating inequality: how high-tech tools profile, police, and punish the poor. First edition. New York, NY: St. Martin’s Press.
López, J. (2020). Experimentando con la pobreza: el SISBÉN y los proyectos de analítica de datos en Colombia. Fundación Karisma. Colombia.
Masiero, S., & Das, S. (2019). Datafying anti-poverty programmes: implications for data justice. Information, Communication & Society, 22(7), 916-933.
Mohamed, S., M. Png & W. Isaac. (2020). Decolonial AI: Decolonial Theory as Sociotechnical Foresight in Artificial Intelligence. Philosophy & Technology. https://doi.org/10.1007/s13347-020-00405-8
Noble, S. U. (2018). Algorithms of oppression: How search engines reinforce racism. New York University Press.
Nobrega, C; Varon, J. (2021). Big tech goes green(washing): feminist lenses to unveil new tools in the master’s house. Giswatch, APC. Available at: https://www.giswatch.org/node/6254
O’Neil, C. (2016). Weapons of Math Destruction: How Big Data Increases Inequality and Threatens Democracy. New York: Crown.
Silva M.R. & Varon, J. (2021). Reconhecimento facial no setor público e identidades trans: tecnopolíticas de controle e ameaça à diversidade de gênero em suas interseccionalidades de raça, classe e território. Coding Rights.
Taylor, L. (2017). What is data justice? The case for connecting digital rights and freedoms globally. Big Data & Society, July-December, 1-14. https://journals.sagepub.com/doi/10.1177/2053951717736335
Tapia, D; Peña. P. (2021). White gold, digital destruction: Research and awareness on the human rights implications of the extraction of lithium perpetrated by the tech industry in Latin American ecosystems. Giswatch, APC. Available at: https://www.giswatch.org/node/6247
Tendayi Achiume, E. (2020). Racial discrimination and emerging digital technologies: a human rights analysis. Report of the Special Rapporteur on contemporary forms of racism, racial discrimination, xenophobia and related intolerance. A/HRC/44/57. Human Rights Council. Forty-fourth session. 15 June–3 July 2020.
West, S.M., Whittaker, M. and Crawford, K. (2019). Discriminating Systems: Gender, Race and Power in AI. AI Now Institute.
Zuboff, S. (2019). The age of surveillance capitalism: the fight for a human future at the new frontier of power. Profile Books.